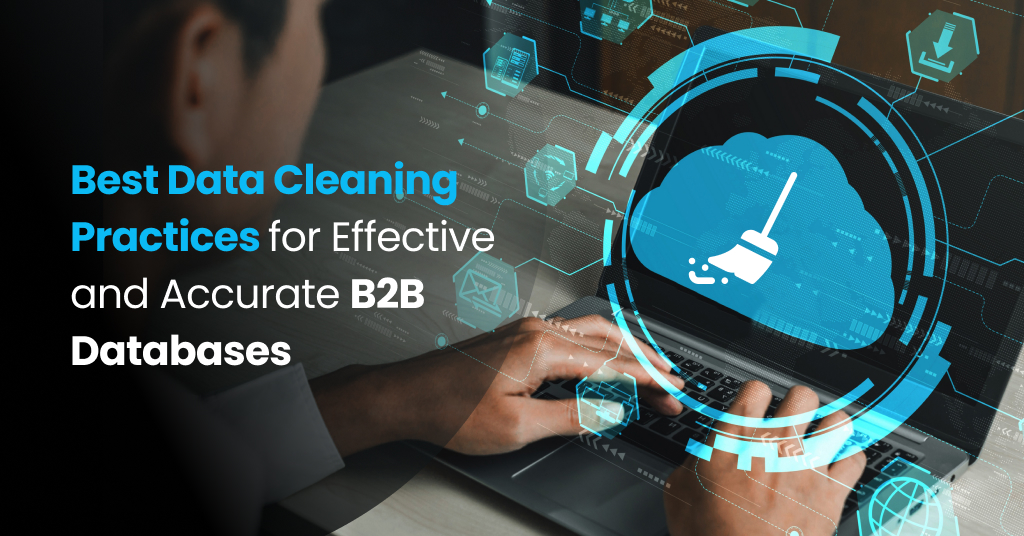
According to Harvard Business Review, almost half of freshly created datasets contain major errors that lead to poor ROI and wastage of time and resources. So, even if you are collecting high-quality data from reliable sources, you must validate & update it regularly for accurate information.
However, maintaining data quality for large datasets is a time and resource-intensive task for businesses. Eliminating errors, duplicacy, and inconsistent formatting and maintaining data hygiene through effective data cleansing practices is crucial for creating high-utility B2B datasets.
To help you out, here are 8 ultimate B2B data cleansing tips you can implement to enhance the accuracy and effectiveness of your datasets.
Common Challenges with Collecting Real-time Data from Various Sources
While collecting data from disparate sources, here are some common issues businesses face regularly:
- data is not structured properly
- missing information
- inaccurate data entries
- irregular format
- data outliers/irrelevant information
- getting errors in uniform data conversion
- data duplicacy
- data placed in wrong fields
The common reasons behind these challenges and data discrepancies are:
- Businesses lacking standardized data entry instructions
- Human errors leading to duplicate or incorrect data
- Retrieving data from inconsistent sources
- Lacking a routine data audit and maintenance
8 Best Data Cleaning Tips for Effective B2B Datasets
To overcome poor data challenges and enhance the accuracy of datasets, here are some data cleansing best practices you can implement:
1. Establish a Data-Quality Plan with Clear Instructions
You must create a data-quality plan to clearly understand what your data looks like (which 27% of data aggregators lack). It helps to establish a realistic baseline for data hygiene.
Determine the data-quality standards per your organization’s needs, including accuracy, completeness, and consistency. By setting up these standards, you will have a clear definition of what makes up clean data and be able to ensure that your data meets these standards.
A well-established data-quality strategy must involve cleaning the datasets before they enter the system along with cleansing real-time data streams, the data source and the destination to save time and effort.
Follow these tips to devise a data-quality strategy for your business :
- Determine the metrics you need to involve in the data-collection method and stick to them.
- Create key performance indicators (KPIs) to check the quality of your data.
- Inspect the data in the initial stages.
- Quickly go through the samples to identify problematic datasets.
- Determine how much effort, time and resources you need to clean specific datasets to avoid over-cleaning.
- First, validate and rectify data thoroughly and then push it into the system.
- Compare new data against previous sets for quality analysis. Ensure they all have the same structure with no irregular patterns.
Make data cleansing a priority for your organization.
2. Regularly Conduct Data Audits
Auditing your data regularly lets you identify any errors or discrepancies at initial levels without wasting further time and resources. It ensures data accuracy by reviewing it for inconsistencies, inaccuracies, and missing information.
You can use tools like Clearbit and ZoomInfo for regular data checkups and cleansing. It ensures you have the correct contact information about prospects. Regular data audits greatly reduce the chances of accumulating poor datasets in large amounts. The fewer flawed datasets you have, the more business you can generate.
3. Automate Data Cleansing with AI/RPA Tools
The next step in maintaining data quality is to identify the errors at the source so it cannot enter into the aggregator database. You can automate this process using AI or RPA-based data cleansing tools. These tools validate data entries at the source level for accuracy and consistency.
But, the technology is ineffective for data outliers where you must make decisions manually.
With RPA and AI-based technologies, you can:
- Cleanse data when it enters the master database, so only standardized information passes down.
- Let only Quality data enter through a Standard Operating Procedure (SOP)
- Improve Data Accuracy with real-time checking and validation of datasets.
- Can merge data collected from multiple sources in a proper format.
- Update and revise the schema to align with the master database.
Automating the data cleansing process can be really effective & convenient for large datasets. You must always validate errors through automated AI/RPA-based tools before sending the data to the warehouse or risk yielding poor results (even with new data).
4. Perform Outliers Detection and Processing
As outliers are unique cases, you must clear them before sending your data to automated tools for further processing. Processing outliers at the initial stage gives you enriched data which improves the efficiency of your marketing campaigns.
There can be various factors responsible for data outliers, such as:
- human error
- system error
- error during data extraction/processing
- dummy outliers used to test detection processes
- due to data mixing from multiple sources
- chances in data
- unexpected mutations/errors in data manipulation
Some common ways to address data outliers are:
- Exploring the data thoroughly or using filters.
- Use visual outliers detection tools & techniques such as TIBCO Spotfire, Z-Score, Winsorized estimators, linear regression, etc.
- Changing or deleting outliers to the datasets
- Check the underlying distribution value before acting upon outliers.
- Analyzing mild outliers in detail
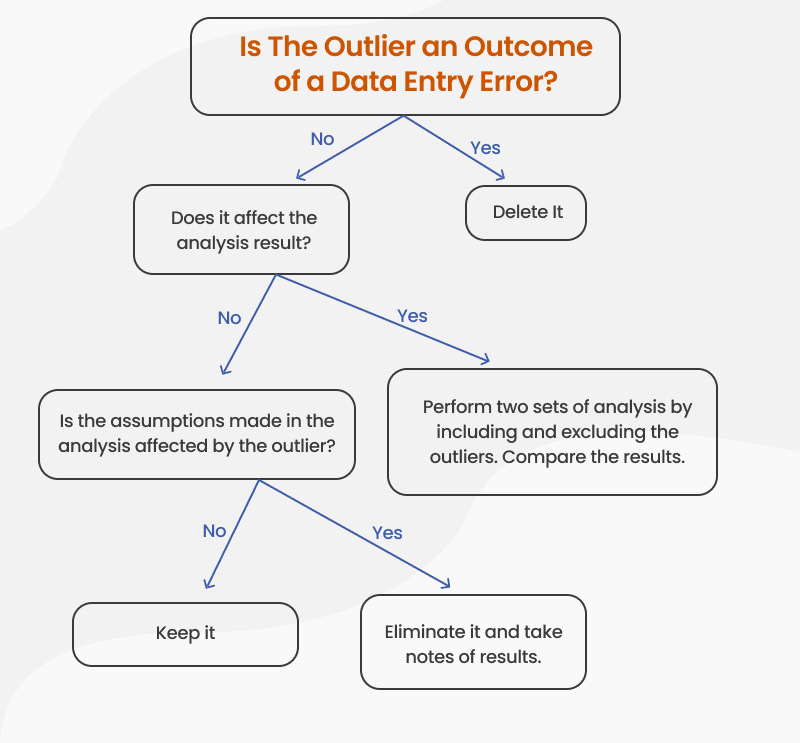
The model and data analyst tools determine whether an outlier needs to be treated as an anomaly. Before changing or deleting the dataset value, it must determine that it won’t affect dataset quality or the cleansing process.
5. Standardize Format Across Datasets
Inconsistent data formats make data management difficult, leading to inaccuracies. Thus you must standardize a format across all datasets for uniformity. Use a consistent format for elements like dates, names, and addresses. To establish a standardized format, determine:
- Whether you want to keep your data in uppercase or lower cases
- The format of date you wish to use
- The measurement units and metrics you wish to use
This makes your data more manageable and easier to work with.
6. Remove Duplicate, Dead, & Irrelevant Entries
Merging data from various sources can lead to data duplicity or inaccuracies. Removing duplicate values ensures you are not double-counting information. Some CRM tools charge businesses for the number of contacts they wish to manage. So, having many duplicates in your dataset can unnecessarily cost you extra.
However, you cannot remove duplicate values arbitrarily from large datasets. You can use advanced data-matching tools to identify such values and then merge/remove them as required.
To make datasets more effective and accurate for better sales results, removing dead/irrelevant entries regularly is crucial.
You can set a specific duration for each entry in the dataset to convert it into sales. Remove the leads that are not responding or converting in that particular duration to save the time and effort of the sales & marketing team.
7. Validate Data Entries for Missing Information
Validating data entries ensure that the data you are entering conforms to your data quality and business standards. It involves:
- Checking data for missing or incorrect information
- Checking and ensuring correct formatting for data
- Regularly update your data to reflect changes in your business, such as new products or services or customer information changes.
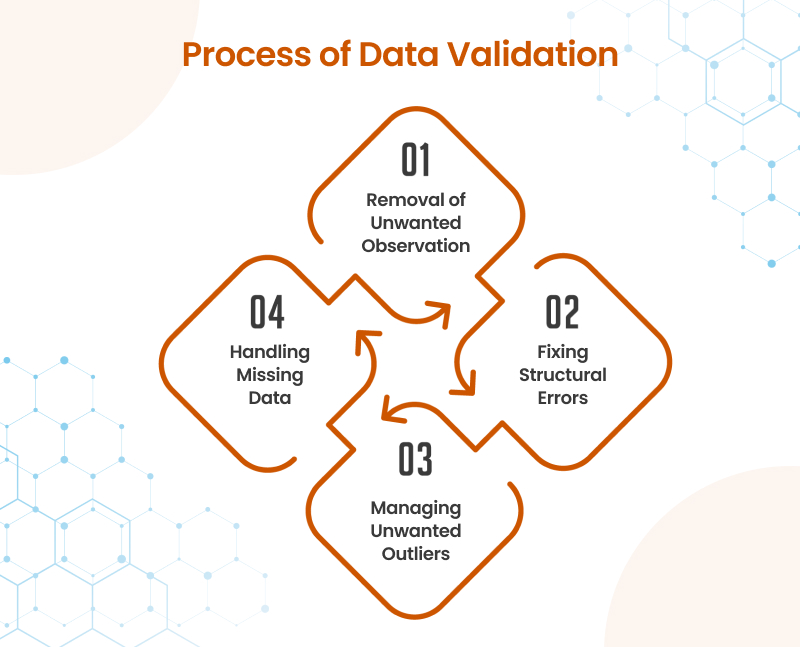
For large datasets, invest in automated tools that smartly detect missing details, incorrect format, etc., to validate entries in no time. Regular validation makes your datasets more accurate, relevant and effective to work.
8. Append Information from Authentic Sources for GDPR/CASL Compliance
The data decay rate varies annually for different industries, organizations and countries. On average, it is between 30%-70% for most sectors and businesses. The information related to your business or prospects keeps changing. Thus you must append the information from authentic sources like LinkedIn, Company Pages, etc., to keep your data up-to-date.
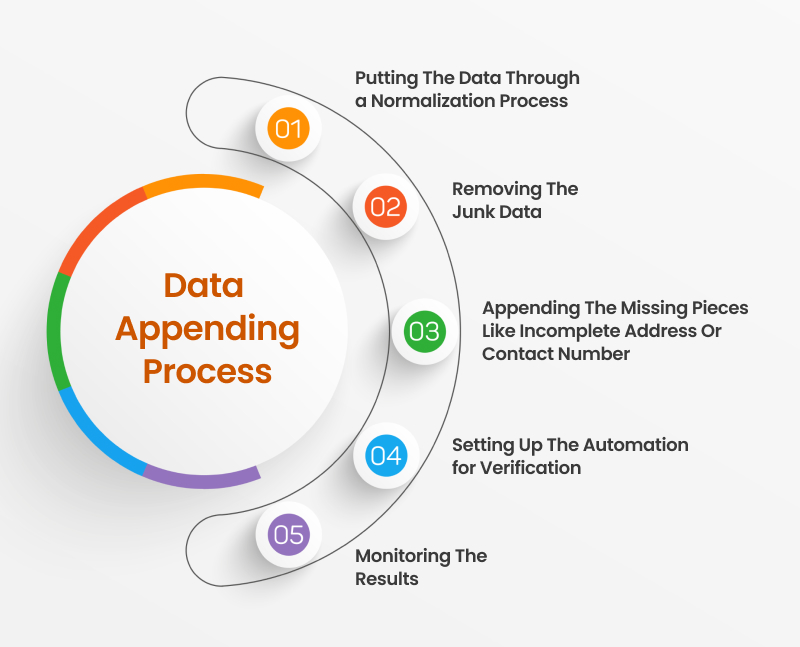
To comply with GDPR or CASL compliance (whichever you follow), your data must be comprehensive and not contain “white spaces”. Keep crucial information such as contact numbers, business addresses, email IDs, and job titles always updated.
Final Verdict!
You cannot prevent bad data from getting in when collecting it from various sources/aggregators. But by following these best practices for data cleaning, you can maintain its quality and effectiveness.
With so many automated data cleansing tools in the market, maintaining your data hygiene has become more convenient. Still, if you want to spend your resources and time only on marketing and sales, hire a reliable B2B data cleansing company that can effectively validate data quality of all your datasets.