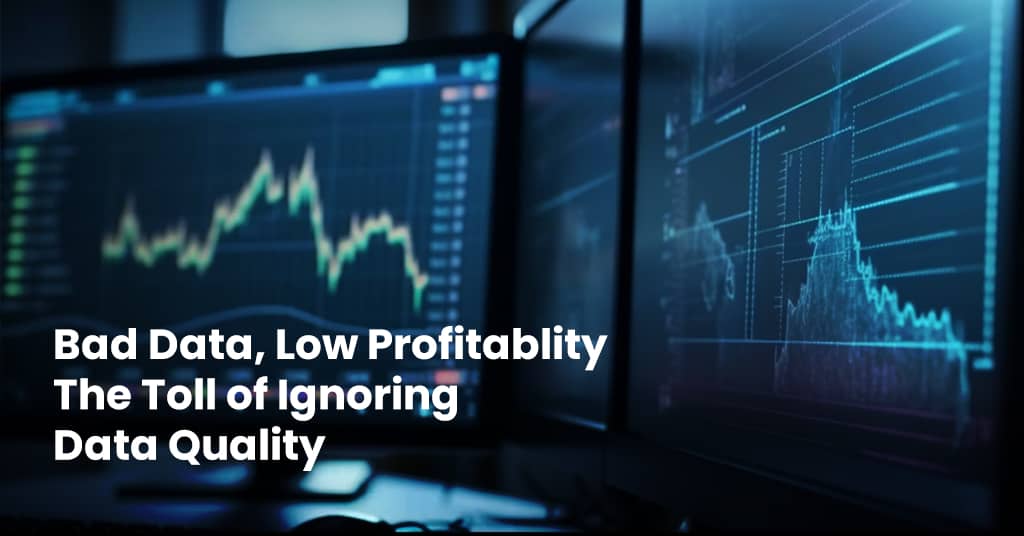
The success of your go-to-market strategies relies on one crucial factor: the quality of your data. Even if you assemble a top-notch sales and marketing team equipped with cutting-edge tools, their efforts will ultimately miss the mark if they are built on the foundation of poor-quality data.
In this blog post, we will explore the detrimental impact of bad data on businesses and the compelling reasons for transitioning from poor-quality to high-quality data. By delving into these aspects, we can better understand the importance of data quality in achieving business success.
What Is Poor Quality Data?
Informally termed as bad or poor data, it refers to information that is inaccurate, irrelevant, misleading, or otherwise unreliable for its intended purpose.
Here’s what low-quality or poor data looks like:
Inaccurate Data
Imagine a customer database that contains incorrect contact information, such as wrong email addresses or phone numbers. Sending marketing campaigns or reaching out to these contacts would be futile, resulting in wasted resources and missed opportunities.
Incomplete Data
Suppose a product inventory system fails to capture essential details like stock levels or variations in product specifications. This incomplete information can lead to errors in order fulfillment, dissatisfied customers, and potential revenue loss.
Inconsistent Data
Inconsistencies arise when different sources or systems use conflicting formats or standards. For instance, one sales report records revenue in USD while another uses a different currency symbol. In addition, misspelled words, unwanted spaces, or casing issues also count for inconsistent data.
Outdated Data
Over time, data can become stale or obsolete. For example, having a mailing list without regularly updating addresses may result in undeliverable mail or communication failures.
Duplicate Data
Duplicate entries occur when the same information is recorded multiple times across different datasets or within the same dataset. This redundancy leads to confusion, inefficient resource allocation, and inaccuracies in reporting.
Key Takeaways: |
|
How Does Data Go Bad?
Data can become inadequate, outdated, inaccurate, or whatever you might like to call it due to several reasons, including but not limited to:
Human Error
Mistakes made during data entry or manual data processing can introduce inaccuracies, inconsistencies, or omissions—for example, typos, incorrect formatting, or misinterpretation of information.
Insufficient Data Validation
Inadequate data quality checks facilitate erroneous or incomplete data entering the system. Without robust validation mechanisms in place, errors can propagate throughout the data lifecycle.
During Legacy Data Migration
When transitioning from old legacy systems to new platforms or during data migration processes, issues such as data loss, data corruption, or data format mismatches can arise, leading to bad data.
Poor Data Integration
Inconsistent data formats, incompatible data structures, or improper data integration practices can introduce inconsistencies and errors when combining data from different sources or systems.
Lack of Data Governance
The absence or weak implementation of data governance practices, including data standards, policies, and controls, can contribute to bad data. Without clear guidelines and oversight, data quality tends to suffer.
Data Decay and Obsolescence
Over time, data can become outdated or irrelevant. Without regular updates or data maintenance processes, businesses may rely on stale information, leading to incorrect decisions or actions based on poor insights.
Inadequate Training and Awareness
Insufficient training or awareness among data users and stakeholders usually results in improper handling or interpretation of data. Additionally, misunderstanding data standards or not abiding by established data protocols can contribute to bad data.
Key Takeaways: |
|
Consequences of Bad Data
Data can either make or break your business. That being said, poorly-kept data can have far-reaching consequences, such as:
- Inaccurate decision-making
- Missed growth opportunities
- Damaged customer relationship
- Decreased operational efficiency
- Increased costs
- Regulatory infractions
Remember, the impact of poor data can vary. For example, the sales team may experience missed cross-selling and upselling opportunities or inefficient targeting. On the other hand, the marketing team may face challenges with lead generation and nurturing or campaign effectiveness. It’s important to recognize that bad data can affect various teams in different manners.
Therefore, to keep your business up and running, you must indulge in data cleansing for an accurate and consistent database.
Let’s see how data cleansing can help you.
Data Cleansing: Protecting Your Business from Costly Errors
Data cleansing or cleaning aims at improving the quality, reliability, and usability of the data, ensuring that it is accurate, consistent, and fit for analysis or operational purposes.
How Is It Done?
Data Audit
The first step involves assessing the data’s completeness, accuracy, and overall quality. This includes examining data sources and formats to understand the context in which the data is used. The goal is to identify any issues or areas that require improvement before proceeding with data scrubbing.
Remove Duplicate and Irrelevant Entries
Once the dataset is audited, the next step is fixing duplicate and irrelevant records to maintain a single, accurate representation of each unique observation. This promotes data integrity and aligns the dataset closely with your intended analysis and business goals.
Handle Missing Data
Yet another crucial step is handling missing or incomplete entries in the database by either entering the missing values or replacing the incomplete entries with correct values based on data requirements and availability.
Filter Unwanted Outliers
Outliers are extreme values that significantly deviate from the majority of the data points. Consider the example:
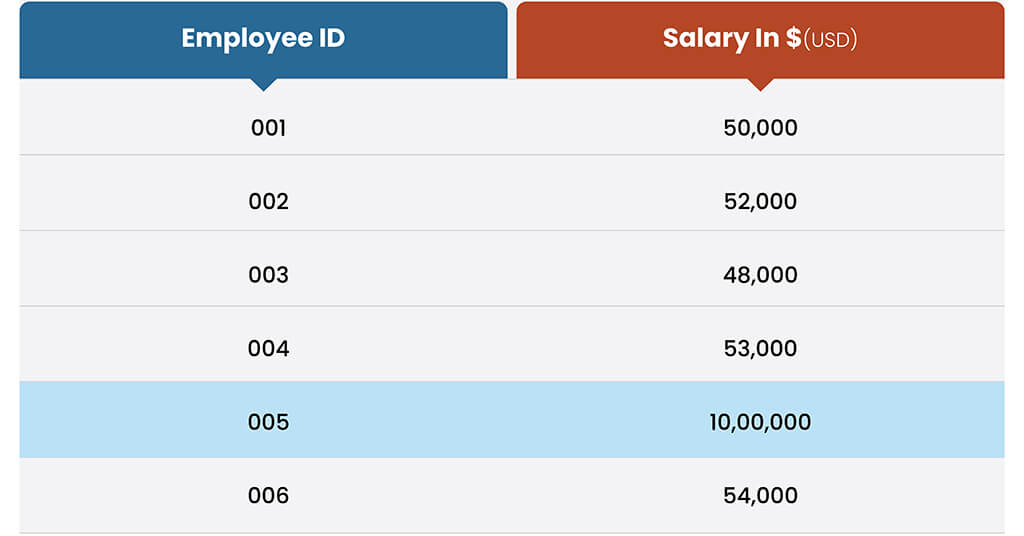
The salary of Employee 005 is significantly higher compared to the other employees. This extreme value of 1,000,000 USD might indicate a data entry error or a unique circumstance that is not representative of the typical salary range.
Such outliers can occur due to measurement errors and data entry mistakes or represent actual but rare occurrences. Depending on the context and analysis goals, handle the outliers by either removing them (if they are erroneous) or adjusting them to minimize their impact on the analysis.
Fix Structural Errors
Next, issues such as inconsistent formatting, incorrect data types, or improper naming conventions are fixed. For instance, inconsistent date formats (“mm/dd/yyyy” vs. “dd-mm-yyyy”) or mixed numeric and string values (see below).
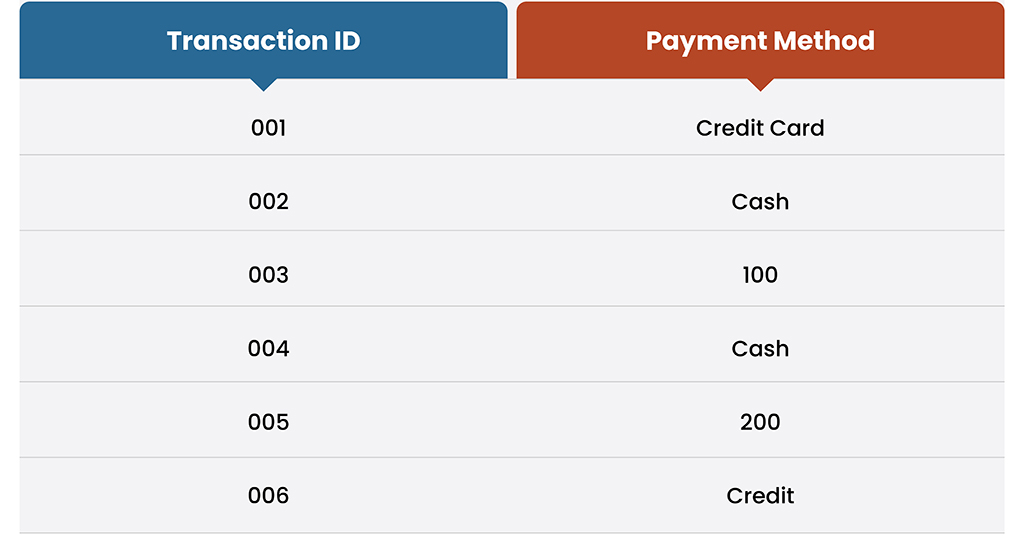
Validate and QA
Once you have dealt with bad-quality data, run the database through rigorous checks to ensure the cleansed data’s accuracy, completeness, and reliability. This includes cross-checking the data against external sources, performing consistency checks, verifying data integrity, and conducting data quality assessments. As one of the most pivotal steps, this ensures all issues are resolved and the resulting data is fit for analysis or decision-making purposes.
The Result
The resultant data, after cleansing, is:
- Valid
- Accurate
- Complete
- Consistent
- Uniform
This data offers businesses a pool of benefits, including
- Accurate decision-making
- Improved business insights
- Enhanced customer relationships
- Increased operational efficiency
- Better targeting and personalization
- Compliance with regulations
- Reduced costs and risks
- Enhanced data-driven strategies
- Increased competitiveness
Move Toward a Clean Database with SunTec India
We can transform your database into a valuable asset with end-to-end data cleansing services. With 20+ years of experience in the industry, we have helped businesses across the globe attain clean, accurate, and comprehensive databases.
See How Our Data Cleansing Experts Helped a Client Boost Their Marketing and Sales Efforts by 52%
With a dedicated data cleansing team, we ensure you get the best-in-class services at affordable prices. Get in touch for a free data audit, and start your journey with us!